Bibcode
Domínguez Sánchez, H.; Martin, G.; Damjanov, I.; Buitrago, F.; Huertas-Company, M.; Bottrell, C.; Bernardi, M.; Knapen, J. H.; Vega-Ferrero, J.; Hausen, R.; Kado-Fong, E.; Población-Criado, D.; Souchereau, H.; Leste, O. K.; Robertson, B.; Sahelices, B.; Johnston, K. V.
Bibliographical reference
Monthly Notices of the Royal Astronomical Society
Advertised on:
5
2023
Citations
11
Refereed citations
6
Description
Interactions between galaxies leave distinguishable imprints in the form of tidal features, which hold important clues about their mass assembly. Unfortunately, these structures are difficult to detect because they are low surface brightness features, so deep observations are needed. Upcoming surveys promise several orders of magnitude increase in depth and sky coverage, for which automated methods for tidal feature detection will become mandatory. We test the ability of a convolutional neural network to reproduce human visual classifications for tidal detections. We use as training ~6000 simulated images classified by professional astronomers. The mock Hyper Suprime Cam Subaru (HSC) images include variations with redshift, projection angle, and surface brightness (μlim = 26-35 mag arcsec-2). We obtain satisfactory results with accuracy, precision, and recall values of Acc = 0.84, P = 0.72, and R = 0.85 for the test sample. While the accuracy and precision values are roughly constant for all surface brightness, the recall (completeness) is significantly affected by image depth. The recovery rate shows strong dependence on the type of tidal features: we recover all the images showing shell features and 87 per cent of the tidal streams; these fractions are below 75 per cent for mergers, tidal tails, and bridges. When applied to real HSC images, the performance of the model worsens significantly. We speculate that this is due to the lack of realism of the simulations, and take it as a warning on applying deep learning models to different data domains without prior testing on the actual data.
Related projects

Spiral Galaxies: Evolution and Consequences
Our small group is well known and respected internationally for our innovative and important work on various aspects of the structure and evolution of nearby spiral galaxies. We primarily use observations at various wavelengths, exploiting synergies that allow us to answer the most pertinent questions relating to what the main properties of
Johan Hendrik
Knapen Koelstra
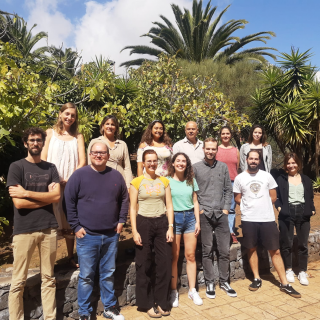
Traces of Galaxy Formation: Stellar populations, Dynamics and Morphology
We are a large, diverse, and very active research group aiming to provide a comprehensive picture for the formation of galaxies in the Universe. Rooted in detailed stellar population analysis, we are constantly exploring and developing new tools and ideas to understand how galaxies came to be what we now observe.
Ignacio
Martín Navarro