Bibcode
Iglesias, F. A.; Cisterna, F.; Sanchez, M.; Machuca, Y.; Lloveras, D.; Manini, F.; Lopez, F. M.; Cremades, H.; Asensio-Ramos, A.
Bibliographical reference
Boletin de la Asociacion Argentina de Astronomia La Plata Argentina
Advertised on:
8
2024
Citations
0
Refereed citations
0
Description
Coronal mass ejections (CMEs) are a major driver of space weather and thus can have important negative technological and social impacts. To assess their geoeffectiveness once they are ejected, it is crucial their prompt identification in coronagraph images. In the last decade, deep neural networks (DNN) have experienced enormous improvements in solving various machine-vision related tasks. One issue when trying to use DNN for CME segmentation, using coronagraph images, is that no large curated dataset exists in the literature that can be used for supervised training. We have produced a synthetic dataset of CME coronagraph images that incorporates the main features of interest, by combining actual quiet (no CME) coronagraph images with synthetic CMEs simulated using the Graduated Cylindrical Shell (GCS) geometric model. In this work, we present preliminary results of a DNN trained to identify and segment the outer envelope of CMEs. This is done by fine-tuning a pre-trained MaskR- CNN model, to produce a GCS-like mask of the CME, present in a single differential coronagraphic image. We compare our results with two other classic CME segmentation algorithms.
Related projects
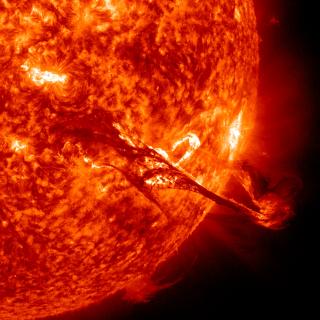
Solar and Stellar Magnetism
Magnetic fields are at the base of star formation and stellar structure and evolution. When stars are born, magnetic fields brake the rotation during the collapse of the mollecular cloud. In the end of the life of a star, magnetic fields can play a key role in the form of the strong winds that lead to the last stages of stellar evolution. During
Tobías
Felipe García