Research led by the Instituto de Astrofísica de Canarias (IAC) has used an innovative technique based on artificial intelligence to study how stars form in galaxies. By analysing 10 000 nearby galaxies, the team have discovered that most stars are born within their own galaxy. Galactic mergers, while important, are not the main source of new stars. Furthermore, the study reveals that more massive galaxies are more affected by these mergers. These results, published in Nature Astronomy, provide new clues about the complex history of galaxies and their evolution over time.
Most galaxies do not exist in isolation but are drawn closer together by gravity and eventually merge. A merger can affect a galaxy in various ways, from increasing its mass and size to transforming its morphology and structure. However, it is not possible to directly determine from observations alone the frequency of mergers in a galaxy’s past or whether these were major events involving galaxies of comparable size or minor ones with smaller galaxies.
Observed differences in the orbits and chemical properties of stellar populations in the Milky Way point towards the distinct cosmic origins of these populations, providing clues about the galaxy’s merging history. However, the number of stars originating from mergers has only been measured for a small number of external galaxies, which highlights the lack of comprehensive studies on the effect of mergers in a statistically significant sample of galaxies.
Cosmological simulations allow us to observe how galaxies evolve across cosmic time, providing details that telescopes cannot capture. Artificial intelligence (AI), on the other hand, is characterised by finding hidden patterns in large data sets with remarkable accuracy. By combining these two powerful tools, it is possible to train a machine learning model on simulated galaxies to predict the properties and cosmic past of real galaxies. This combination of simulations and AI represents a significant advance in our ability to study the universe.
Galactic mergers more common in massive galaxies
Using this approach, an IAC team has analysed 10 000 nearby galaxies to determine what proportion of their stars formed from mergers with other galaxies. The study has revealed that only 20% of the stellar mass in present day galaxies is the result of past mergers, so most of the stars in today's Universe formed internally.
According to the study, while galaxies with low stellar masses form almost all of their stars internally, the most massive galaxies obtain up to 35-40% of their stellar mass through mergers. These findings suggest that more massive galaxies are more profoundly impacted by mergers than less massive ones. Similarly, old, elliptical galaxies, and galaxies that no longer form new stars have accreted a higher fraction of their stars than their spiral and star-forming counterparts.
Additionally, the study also shows that galaxies at the center of more massive dark matter halos tend to have experienced more substantial mergers than those centered in less massive halos, suggesting that a galaxy’s cosmic environment can influence its merging history.
“The ability to explore the merging history of galaxies could help to better understand how galaxies form and evolve” explains Eirini Angeloudi, a PhD student at the IAC who led the study. “For example, one can investigate whether mergers contribute to the creation of specific types of galaxies or set in motion vital processes, such as intense bursts of star-formation or fuelling the activity of Active Galactic Nuclei.”
“Our work demonstrates how cosmological simulations and machine learning can be combined to provide a tool to gain information about the cosmic past of present-day galaxies. This technique could be extended to construct complete merging trees of galaxies” says Marc Huertas, a researcher at the IAC and co-author of the study.
Next challenge: more distant galaxies
Despite the promising results, the team notes that the simulations used to study the Universe are limited by what we know so far. This means they can be inaccurate and flawed, especially when trying to understand complex phenomena on small scales.
“Although we have identified correlations between the properties of a galaxy and the effect of mergers on its stellar mass, correlation does not always imply causation” says Jesús Falcón, a researcher at the IAC who has participated in the research. “Therefore, we cannot say with confidence whether mergers are the primary factors behind the various processes observed in nearby galaxies, such as morphological transformations or the quenching of star formation.”
As a next step, the team plans to extend this investigation to more distant galaxies, which would make it possible to trace the progression of merging events and assess their contribution to a galaxy’s stellar mass across different evolutionary stages of the Universe. “Such advances would provide insight into how galaxy mergers affect the evolution of galaxies over cosmic time, shedding light on their role in shaping the diversity of galactic structures and properties observed today” concludes Angeloudi.
Article: Angeloudi, E., Falcón-Barroso, J., Huertas-Company, M. et al. Constraints on the in situ and ex situ stellar masses in nearby galaxies obtained with artificial intelligence. Nat Astron (2024). https://doi.org/10.1038/s41550-024-02327-3
Contact at the IAC:
Eirini Angeloudi, eirini.angeloudi [at] iac.es (eirini[dot]angeloudi[at]iac[dot]es)
Marc Huertas Company, mhuertas [at] iac.es (mhuertas[at]iac[dot]es)
Jesús Falcón Barroso, jfalcon [at] iac.es (jfalcon[at]iac[dot]es)
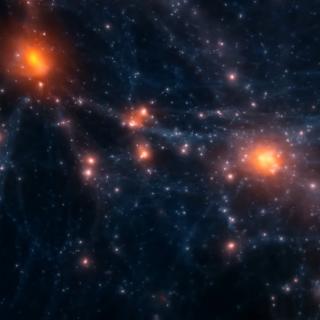
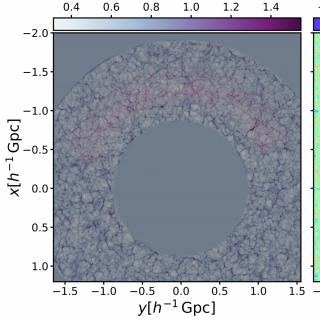
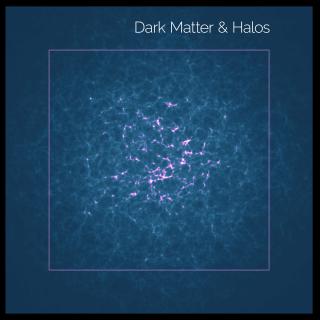