Bibcode
Walker, A. R.; Zuntz, J.; Thomas, D.; Swanson, M. E. C.; Tarle, G.; Suchyta, E.; Soares-Santos, M.; Sobreira, F.; Smith, R. C.; Smith, M.; Schubnell, M.; Schindler, R.; Scarpine, V.; Sanchez, E.; Plazas, A. A.; Nord, B.; Miquel, R.; Menanteau, F.; Melchior, P.; March, M.; Maia, M. A. G.; Lahav, O.; Kuehn, K.; Kuropatkin, N.; James, D. J.; Hoyle, B.; Hollowood, D. L.; Honscheid, K.; Gutierrez, G.; Hartley, W. G.; Gschwend, J.; Gruendl, R. A.; Gruen, D.; Gerdes, D. W.; Gaztanaga, E.; García-Bellido, J.; Frieman, J.; Fosalba, P.; Evrard, A. E.; Doel, P.; De Vicente, J.; Davis, C.; da Costa, L. N.; D'Andrea, C. B.; Cunha, C. E.; Carretero, J.; Carrasco Kind, M.; Carnero Rosell, A.; Buckley-Geer, E.; Avila, S.; Brooks, D.; Annis, J.; Abbott, T. M. C.; Abdalla, F. B.; Fischer, J. L.; Kaviraj, S.; Bernardi, M.; Domínguez Sánchez, H.; Huertas-Company, M.
Referencia bibliográfica
Monthly Notices of the Royal Astronomical Society, Volume 484, Issue 1, p.93-100
Fecha de publicación:
3
2019
Número de citas
71
Número de citas referidas
63
Descripción
Deep learning (DL) algorithms for morphological classification of
galaxies have proven very successful, mimicking (or even improving)
visual classifications. However, these algorithms rely on large training
samples of labelled galaxies (typically thousands of them). A key
question for using DL classifications in future Big Data surveys is how
much of the knowledge acquired from an existing survey can be exported
to a new data set, i.e. if the features learned by the machines are
meaningful for different data. We test the performance of DL models,
trained with Sloan Digital Sky Survey (SDSS) data, on Dark Energy Survey
(DES) using images for a sample of ˜5000 galaxies with a similar
redshift distribution to SDSS. Applying the models directly to DES data
provides a reasonable global accuracy (˜90 per cent), but small
completeness and purity values. A fast domain adaptation step,
consisting of a further training with a small DES sample of galaxies
(˜500-300), is enough for obtaining an accuracy >95 per cent
and a significant improvement in the completeness and purity values.
This demonstrates that, once trained with a particular data set,
machines can quickly adapt to new instrument characteristics (e.g. PSF,
seeing, depth), reducing by almost one order of magnitude the necessary
training sample for morphological classification. Redshift evolution
effects or significant depth differences are not taken into account in
this study.
Proyectos relacionados
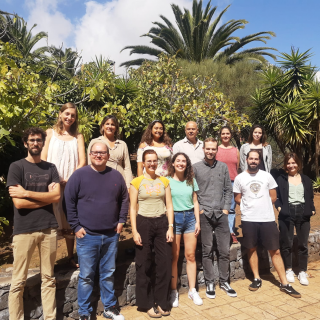
Huellas de la Formación de las Galaxias: Poblaciones estelares, Dinámica y Morfología
Bienvenida a la página web del g rupo de investigación Traces of Galaxy Formation. Somos un grupo de investigación amplio, diverso y muy activo cuyo objetivo principal es entender la formación de galaxias en el Universo de una manera lo más completa posible. Con el estudio detellado de las poblaciones estelares como bandera, estamos constantemente
Ignacio
Martín Navarro